Voiced by Amazon Polly |
Overview
In today’s fast-paced digital world, businesses are inundated with vast amounts of data from various sources. Extracting meaningful insights from this data is crucial for making informed decisions, identifying trends, and detecting anomalies that could signify potential issues or opportunities. Azure Anomaly Detector, a powerful tool offered by Microsoft Azure, is at the forefront of enabling organizations to harness the power of machine learning to detect anomalies in their data streams accurately. In this blog, we’ll delve into the capabilities of Azure Anomaly Detector, its applications across different industries, and how it is revolutionizing how businesses leverage data analytics.
Pioneers in Cloud Consulting & Migration Services
- Reduced infrastructural costs
- Accelerated application deployment
Introduction
Azure Anomaly Detector is a part of Microsoft’s suite of AI-powered tools and services hosted on the Azure cloud platform. It utilizes advanced machine learning algorithms to analyze time-series data and identify anomalies, deviations, or outliers within the data. These anomalies could represent unusual patterns, unexpected spikes or drops, or deviations from the norm, which may indicate potential issues, fraud, or emerging trends.
It employs supervised and unsupervised learning, statistical modeling, and probabilistic algorithms to continuously learn from data streams and improve anomaly detection accuracy over time.
Applications Across Industries
Azure Anomaly Detector finds applications across various industries where detecting anomalies in data streams is critical for ensuring operational efficiency, detecting fraud, preventing equipment failures, optimizing resource utilization, and enhancing customer experiences. Let’s explore some industry-specific use cases:
- Finance and Banking:
In the finance sector, Azure Anomaly Detector can detect unusual patterns in financial transactions, identify fraudulent activities such as unauthorized transactions or account takeovers, and monitor stock market fluctuations. By detecting anomalies in real-time, financial institutions can mitigate risks, protect customers’ assets, and maintain regulatory compliance.
- Manufacturing and IoT:
In manufacturing and IoT (Internet of Things) environments, Azure Anomaly Detector can analyze sensor data from machinery and equipment to identify anomalies that may indicate potential equipment failures or maintenance issues. Manufacturers can optimize production processes, reduce maintenance costs, and ensure smooth operations by predicting and preventing equipment downtime.
- Retail and E-commerce:
In retail and e-commerce, Azure Anomaly Detector can analyze sales data to detect anomalies in purchasing patterns, identify trends, and predict demand fluctuations. Retailers can use this information to optimize inventory management, personalize marketing campaigns, and enhance the overall shopping experience for customers.
- Healthcare:
In the healthcare industry, Azure Anomaly Detector can analyze patient data, such as vital signs or medical sensor readings, to detect anomalies indicating health complications or deviations from normal health conditions. Healthcare providers can use this information for early detection of diseases, patient monitoring, and improving clinical decision-making.
Leveraging Azure Anomaly Detector for Business Insights
Integrating Azure Anomaly Detector into existing data analytics workflows is relatively straightforward, thanks to its seamless integration with other Azure services and APIs. Here are some steps businesses can take to leverage Azure Anomaly Detector effectively:
- Data Preparation: Prepare the time-series data by cleaning, formatting, and structuring it appropriately for analysis. Ensure the data contains relevant features and attributes to help identify anomalies effectively.
- Model Training: Train the Azure Anomaly Detector model using historical data to learn normal patterns and behaviors. Choose an appropriate anomaly detection algorithm and fine-tune model parameters based on the specific requirements of the use case.
- Real-time Monitoring: Deploy the trained model to monitor real-time data streams and detect anomalies as they occur. Set up alerts or notifications to notify stakeholders whenever anomalies are detected, enabling timely intervention and decision-making.
- Continuous Improvement: Continuously monitor model performance and retrain the model periodically using updated data to adapt to changing patterns and ensure accurate anomaly detection over time. Incorporate feedback from stakeholders to refine the model and improve its effectiveness.
Conclusion
Azure Anomaly Detector represents a significant advancement in data analytics, empowering businesses across industries to effectively detect and respond to anomalies in their data streams. By leveraging the power of machine learning and cloud computing, Azure Anomaly Detector enables organizations to uncover valuable insights, mitigate risks, and drive informed decision-making. As businesses increasingly rely on data-driven strategies to gain a competitive edge, Azure Anomaly Detector emerges as a valuable tool for unlocking the full potential of their data assets and driving innovation in the digital age.
Drop a query if you have any questions regarding Azure Anomaly Detector and we will get back to you quickly.
Empowering organizations to become ‘data driven’ enterprises with our Cloud experts.
- Reduced infrastructure costs
- Timely data-driven decisions
About CloudThat
CloudThat is a leading provider of Cloud Training and Consulting services with a global presence in India, the USA, Asia, Europe, and Africa. Specializing in AWS, Microsoft Azure, GCP, VMware, Databricks, and more, the company serves mid-market and enterprise clients, offering comprehensive expertise in Cloud Migration, Data Platforms, DevOps, IoT, AI/ML, and more.
CloudThat is recognized as a top-tier partner with AWS and Microsoft, including the prestigious ‘Think Big’ partner award from AWS and the Microsoft Superstars FY 2023 award in Asia & India. Having trained 650k+ professionals in 500+ cloud certifications and completed 300+ consulting projects globally, CloudThat is an official AWS Advanced Consulting Partner, Microsoft Gold Partner, AWS Training Partner, AWS Migration Partner, AWS Data and Analytics Partner, AWS DevOps Competency Partner, Amazon QuickSight Service Delivery Partner, Amazon EKS Service Delivery Partner, AWS Microsoft Workload Partners, Amazon EC2 Service Delivery Partner, and many more.
To get started, go through our Consultancy page and Managed Services Package, CloudThat’s offerings.
FAQs
1. Can Azure Anomaly Detector be used for anomaly detection in non-traditional datasets?
ANS: – Yes, Azure Anomaly Detector is highly versatile and can be applied to a wide range of datasets beyond the typical time-series data. It can analyze text data, images, and audio streams to detect anomalies or deviations from expected patterns. For example, it can identify unusual patterns in customer reviews, detect anomalies in satellite imagery, or analyze voice recordings for anomalies in speech patterns.
2. How does Azure Anomaly Detector handle seasonality and periodic patterns in data?
ANS: – Azure Anomaly Detector employs sophisticated algorithms capable of identifying and adapting to seasonality and periodic patterns present in the data. It automatically learns the underlying patterns and trends in the data, distinguishing between normal fluctuations and true anomalies. By incorporating seasonality detection mechanisms, Azure Anomaly Detector can accurately detect anomalies even in datasets with strong seasonal components, such as sales data or weather patterns.
3. Can Azure Anomaly Detector detect anomalies in streaming data in real-time?
ANS: – Yes, Azure Anomaly Detector is designed to analyze streaming data in real-time, making it well-suited for applications requiring timely anomaly detection and response. By integrating with Azure Stream Analytics or other real-time data processing services, Azure Anomaly Detector can continuously monitor incoming data streams, detect anomalies, and trigger real-time alerts or actions. This capability is particularly valuable for industries such as finance, healthcare, and manufacturing, where timely detection of anomalies is critical.
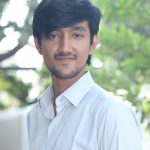
WRITTEN BY Modi Shubham Rajeshbhai
Shubham Modi is working as a Research Associate - Data and AI/ML in CloudThat. He is a focused and very enthusiastic person, keen to learn new things in Data Science on the Cloud. He has worked on AWS, Azure, Machine Learning, and many more technologies.
Comments