Voiced by Amazon Polly |
Overview
In today’s data-driven world, businesses and researchers constantly seek innovative ways to extract meaningful insights from vast datasets. One of the most promising technologies at the forefront of data analysis is Generative Artificial Intelligence (AI). This advanced field combines machine learning and deep learning capabilities to create synthetic data, generate realistic predictions, and enhance decision-making. This blog post will explore how Generative AI revolutionizes data analysis and uncover its potential applications in various industries.
Pioneers in Cloud Consulting & Migration Services
- Reduced infrastructural costs
- Accelerated application deployment
Introduction
Generative AI is a branch of artificial intelligence that generates new data instances that exhibit similarities to a provided dataset. Unlike traditional AI models that perform tasks based on pre-defined rules or patterns, Generative AI can generate new, unique data points that follow the statistical patterns and structures of the training data. This capability makes it incredibly valuable in data analysis.
Use cases of Generative AI for Data Analysis
- Data Augmentation – Data augmentation is one of the primary applications of generative AI in data analysis. You can expand your dataset and enhance its diversity by leveraging generative models. For instance, if you have a limited dataset for sentiment analysis, generative AI can create additional text samples, thereby improving the robustness of your analysis.
- Anomaly Detection – Generative AI can be employed for anomaly detection in data. Training a generative model on normal data samples can flag any data point that deviates significantly from the generated distribution as an anomaly. This technique is particularly valuable in cybersecurity, fraud detection, and quality control.
- Time-Series Forecasting – Time-series data analysis often involves predicting future values based on historical data. Generative AI models can produce various potential future scenarios, thereby assisting analysts in making well-informed decisions and preparing for unforeseen circumstances.
- Natural Language Processing (NLP) Applications – In natural language processing, generative AI shines brightly. It can be employed for various data analysis tasks, such as:
- Text Summarization: Generating concise summaries of lengthy documents or articles.
- Data Labelling: Automatically generating labels or tags for textual data.
- Content Generation: Creating product descriptions, reviews, or social media posts for marketing analysis.
- Image Data Analysis – Generative AI is not limited to text data and is also incredibly powerful in analyzing and generating images. For instance:
- Image Denoising: Removing noise from images can be essential for image analysis in medical imaging or satellite imagery.
- Style Transfer: Changing the artistic style of images for creative data visualization.
- Facial Data Augmentation: Generating additional facial images to improve facial recognition models.
Commonly Used Generative AI Tools and Frameworks for Data Analysis
Conclusion
However, using Generative AI responsibly is crucial, addressing privacy concerns and continuously improving model robustness to maximize its benefits.
Drop a query if you have any questions regarding Generative AI model for Data Analysis and we will get back to you quickly.
Empowering organizations to become ‘data driven’ enterprises with our Cloud experts.
- Reduced infrastructure costs
- Timely data-driven decisions
About CloudThat
CloudThat is an official AWS (Amazon Web Services) Advanced Consulting Partner and Training partner, AWS Migration Partner, AWS Data and Analytics Partner, AWS DevOps Competency Partner, Amazon QuickSight Service Delivery Partner, AWS EKS Service Delivery Partner, and Microsoft Gold Partner, helping people develop knowledge of the cloud and help their businesses aim for higher goals using best-in-industry cloud computing practices and expertise. We are on a mission to build a robust cloud computing ecosystem by disseminating knowledge on technological intricacies within the cloud space. Our blogs, webinars, case studies, and white papers enable all the stakeholders in the cloud computing sphere.
To get started, go through our Consultancy page and Managed Services Package, CloudThat’s offerings.
FAQs
1. Can Generative AI be used for anomaly detection in data analysis?
ANS: – Yes, Generative AI can model the normal distribution of data and identify any data points that deviate significantly from this learned distribution as anomalies. This is valuable for fraud detection, quality control, and outlier detection.
2. What role does Generative AI play in anomaly detection?
ANS: – Generative AI can learn the normal distribution of data and identify anomalies or outliers by flagging data points that deviate significantly from the learned distribution. This is valuable for fraud detection and quality control.
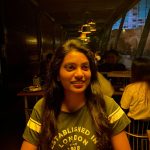
WRITTEN BY Anusha R
Anusha R is a Research Associate at CloudThat. She is interested in learning advanced technologies and gaining insights into new and upcoming cloud services, and she is continuously seeking to expand her expertise in the field. Anusha is passionate about writing tech blogs leveraging her knowledge to share valuable insights with the community. In her free time, she enjoys learning new languages, further broadening her skill set, and finds relaxation in exploring her love for music and new genres.
Comments