Voiced by Amazon Polly |
Introduction
Amazon SageMaker Canvas has introduced a groundbreaking feature that enables the deployment of machine learning (ML) models to real-time inferencing endpoints. This advancement empowers users to seamlessly transition their ML models into production, facilitating immediate action based on the insights derived from ML predictions.
With this capability, Amazon SageMaker Canvas becomes a versatile tool for driving actionable decisions and leveraging the power of ML in a user-friendly and accessible manner.
Pioneers in Cloud Consulting & Migration Services
- Reduced infrastructural costs
- Accelerated application deployment
Advantages of Deploying to Amazon SageMaker Real-Time Endpoints
- Real-Time Predictive Power: Models deployed to real-time endpoints can instantly process data streams, providing prompt insights for informed decision-making.
- Scalability to Match Demand: Amazon SageMaker real-time endpoints exhibit flexibility by scaling up or down to handle varying workloads, ensuring consistent performance during peak traffic.
- Comprehensive Monitoring and Observability: Amazon SageMaker real-time endpoints offer detailed monitoring and observability features, allowing users to track model performance, identify anomalies, and optimize model behavior.
- Integration with Existing Production Systems: Effortless integration with existing production systems enables the seamless incorporation of ML insights into business processes.
Prerequisites for Deployment
Before initiating the deployment process, ensure the following prerequisites are met:
- Amazon SageMaker Canvas Admin Permissions: Grant the necessary permissions for deploying models to Amazon SageMaker endpoints, managed within the Amazon SageMaker domain hosting the Amazon SageMaker Canvas application.
- Model Version Approval: Obtain approval for the deployment of the ML model version from an authorized user, ensuring only thoroughly evaluated, high-quality models enter production.
Step-by-Step Deployment Process
Follow these straightforward steps to deploy an ML model built-in Amazon SageMaker Canvas to an Amazon SageMaker real-time endpoint:
- Accessing SageMaker Canvas: Log in to your Amazon SageMaker Canvas workspace using AWS credentials.
- Locating the Model: Navigate to the model intended for deployment, having undergone training, evaluation, and approval.
- Initiating Deployment: Click the “Deploy” button associated with the chosen model to launch the deployment wizard.
- Configuring Deployment Settings: Specify deployment details, including the model version, instance type, and endpoint configuration.
- Review and Confirmation: Carefully review deployment settings to ensure accuracy.
- Deploying the Model: Click the “Deploy” button to initiate the deployment process, create a Amazon SageMaker real-time endpoint, and deploy the selected model version.
Monitoring and Managing Deployed Models
Effectively monitor and manage deployed models using Amazon SageMaker Studio or the AWS Management Console, providing real-time insights into model performance, resource utilization, and endpoint health.
Revolutionizing ML Adoption
Deploying ML models from Amazon SageMaker Canvas to Amazon SageMaker real-time endpoints empowers businesses to integrate ML-driven insights into production seamlessly. This democratization of ML allows a broader user base to harness its power for real-time decision-making and business optimization.
Conclusion
The incorporation of Amazon SageMaker Canvas and its advanced deployment functionality for real-time inferencing endpoints represents a substantial advancement in the operationalization of machine learning models. This innovation facilitates a smooth production transition, allowing instant on-demand predictions to derive actionable insights. Amazon SageMaker Canvas, with its user-friendly, no-code interface, serves the needs of analysts and citizen data scientists, streamlining the application of machine learning in practical scenarios efficiently and making it accessible. This unique capability positions Amazon SageMaker Canvas as a versatile tool for influencing informed decisions and generating impactful outcomes through the potential of machine learning.
Drop a query if you have any questions regarding Amazon SageMaker Canvas and we will get back to you quickly.
Making IT Networks Enterprise-ready – Cloud Management Services
- Accelerated cloud migration
- End-to-end view of the cloud environment
About CloudThat
CloudThat is an official AWS (Amazon Web Services) Advanced Consulting Partner and Training partner, AWS Migration Partner, AWS Data and Analytics Partner, AWS DevOps Competency Partner, Amazon QuickSight Service Delivery Partner, Amazon EKS Service Delivery Partner, Microsoft Gold Partner, AWS Microsoft Workload Partners, Amazon EC2 Service Delivery Partner, and many more, helping people develop knowledge of the cloud and help their businesses aim for higher goals using best-in-industry cloud computing practices and expertise. We are on a mission to build a robust cloud computing ecosystem by disseminating knowledge on technological intricacies within the cloud space. Our blogs, webinars, case studies, and white papers enable all the stakeholders in the cloud computing sphere.
To get started, go through our Consultancy page and Managed Services Package, CloudThat’s offerings.
FAQs
1. What is the significance of deploying ML models built in Amazon SageMaker Canvas to Amazon SageMaker real-time endpoints?
ANS: – Deploying ML models from Amazon SageMaker Canvas to Amazon SageMaker real-time endpoints is crucial as it allows users to seamlessly integrate their models into production environments. This ensures that the ML models can process data in real time, providing immediate predictions for timely decision-making.
2. How does the no-code nature of Amazon SageMaker Canvas contribute to the deployment process?
ANS: – Amazon SageMaker Canvas, a no-code platform, simplifies the deployment process for users without extensive coding expertise. It enables analysts and citizen data scientists to deploy ML models effortlessly, expanding the accessibility of machine learning capabilities to a broader audience.
3. What benefits do Amazon SageMaker real-time endpoints offer for deployed ML models?
ANS: – Amazon SageMaker real-time endpoints provide several advantages, including the ability to process data streams in real-time, scalability to handle varying workloads, comprehensive monitoring features for tracking model performance, and seamless integration with existing production systems.
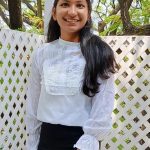
WRITTEN BY Aayushi Khandelwal
Aayushi, a dedicated Research Associate pursuing a Bachelor's degree in Computer Science, is passionate about technology and cloud computing. Her fascination with cloud technology led her to a career in AWS Consulting, where she finds satisfaction in helping clients overcome challenges and optimize their cloud infrastructure. Committed to continuous learning, Aayushi stays updated with evolving AWS technologies, aiming to impact the field significantly and contribute to the success of businesses leveraging AWS services.
Comments