Voiced by Amazon Polly |
Introduction
Apache Spark has become a go-to framework for big data processing, providing a fast and general-purpose cluster-computing system. Databricks, a unified analytics platform built on top of Apache Spark, enhances the capabilities of Spark and makes it easier to deploy, manage, and scale. However, optimizing Spark jobs becomes crucial for maintaining performance and cost-effectiveness as data grows. In this blog, we will explore various strategies for optimizing Spark jobs in the context of Databricks.
Pioneers in Cloud Consulting & Migration Services
- Reduced infrastructural costs
- Accelerated application deployment
Understanding the Basics
- Data Partitioning
Data partitioning is an important factor in Spark job optimization. By partitioning data across multiple partitions, Spark can distribute the workload across multiple executor nodes, improving parallelism and reducing processing time.
- Partitioning Strategy: The partitioning strategy should be based on the characteristics of the data and the Spark job. For example, a job that involves aggregating data by a specific column might benefit from partitioning the data by that column.
- Partition Size: The partition size should be large enough for efficient data processing but small enough to avoid shuffle operations. Too many small partitions can lead to excessive overhead, while too few large partitions can limit parallelism.
- Caching and Persistence
Caching frequently accessed DataFrames or RDDs can reduce the need for recomputation, leading to performance improvements. Databricks simplifies this process with its caching capabilities, allowing you to persist intermediate results and reuse them across multiple stages.
- Data Skewing
Data skewness, where certain partitions have significantly more data than others, can lead to performance bottlenecks. Databricks offers tools for identifying and addressing skewed data, such as the spark.sql.shuffle.partitions
configuration and the spark.sql.adaptive.skewJoin.enabled
option.
Databricks-Specific Optimizations
- Auto-Optimization
Databricks provides built-in features for automatic optimization. The Auto Optimize option intelligently tunes configurations based on the characteristics of your Spark job, optimizing resources like executor memory, shuffle partitions, and broadcast join thresholds.
- Delta Lake
Delta Lake, an open-source storage layer for big data workloads, is tightly integrated with Databricks. Leveraging Delta Lake for storage can enhance performance by enabling features like schema evolution, ACID transactions, and optimized data skipping for faster queries.
Performance Tuning Strategies
- Shuffle Tuning
Shuffle operations can be resource-intensive. Databricks allows you to monitor and optimize shuffle operations using the UI and Spark’s dynamic allocation features. Adjusting parameters like spark.shuffle.file.buffer
and spark.reducer.maxSizeInFlight
can mitigate shuffle-related performance issues.
- Broadcast Joins
Databricks supports broadcast joins, a technique where smaller DataFrames are broadcasted to all nodes, reducing the need for shuffling. Properly configuring the spark.sql.autoBroadcastJoinThreshold
parameter is crucial for optimizing the size threshold for broadcasting.
- Cluster Configuration
The cluster configuration plays a crucial role in Spark job performance. It determines the number of resources available to the Spark application, such as the number of executor nodes, the amount of memory per executor, and the type of instance used for each node. Choosing the right cluster configuration is essential to balance cost and performance.
- Cluster Size: The cluster size should be based on the size of the data being processed and the complexity of the Spark job. A larger cluster can handle larger datasets and more complex jobs, but it also comes with a higher cost.
- Instance Type: The instance type determines the CPU, memory, and storage amount available to each executor node. Choosing the right instance type can significantly impact performance. For instance, a memory-intensive job may benefit from more memory-intensive instances, while a CPU-intensive job may require more CPU cores.
- Storage: The storage configuration determines where Spark stores intermediate data and shuffle files. Using SSDs for storage can significantly improve performance, especially for shuffle-heavy jobs.
Monitoring and Troubleshooting
Monitoring Spark job performance and identifying bottlenecks is essential for optimization. Databricks provides various tools for monitoring Spark jobs, including the Spark UI and Databricks Job History.
- Spark UI: The Spark UI provides detailed information about the execution of a Spark job, including each stage’s execution time, memory usage, and shuffle data size.
- Databricks Job History: Databricks Job History stores historical Spark job metrics, allowing you to track performance trends and identify patterns.
Conclusion
Regular monitoring, diagnostics, and using Databricks’ built-in tools empower you to optimize and fine-tune your Spark applications for maximum efficiency continually.
Drop a query if you have any questions regarding Spark jobs and we will get back to you quickly.
Empowering organizations to become ‘data driven’ enterprises with our Cloud experts.
- Reduced infrastructure costs
- Timely data-driven decisions
About CloudThat
CloudThat is a leading provider of Cloud Training and Consulting services with a global presence in India, the USA, Asia, Europe, and Africa. Specializing in AWS, Microsoft Azure, GCP, VMware, Databricks, and more, the company serves mid-market and enterprise clients, offering comprehensive expertise in Cloud Migration, Data Platforms, DevOps, IoT, AI/ML, and more.
CloudThat is recognized as a top-tier partner with AWS and Microsoft, including the prestigious ‘Think Big’ partner award from AWS and the Microsoft Superstars FY 2023 award in Asia & India. Having trained 650k+ professionals in 500+ cloud certifications and completed 300+ consulting projects globally, CloudThat is an official AWS Advanced Consulting Partner, AWS Training Partner, AWS Migration Partner, AWS Data and Analytics Partner, AWS DevOps Competency Partner, Amazon QuickSight Service Delivery Partner, Amazon EKS Service Delivery Partner, Microsoft Gold Partner, AWS Microsoft Workload Partners, Amazon EC2 Service Delivery Partner, and many more.
To get started, go through our Consultancy page and Managed Services Package, CloudThat’s offerings.
FAQs
1. How does Spark handle data processing, and what are RDDs?
ANS: – Spark processes data using Resilient Distributed Datasets (RDDs), distributed data collections across a cluster. RDDs are immutable and fault-tolerant, allowing Spark to recover from node failures efficiently.
2. How does auto-scaling work in Databricks, and what benefits does it offer for Spark clusters?
ANS: – Databricks leverages auto-scaling to adjust the number of worker nodes in a Spark cluster dynamically based on the current workload. This adaptive scaling ensures optimal resource utilization and cost efficiency, especially in cloud environments where resources are billed based on usage.
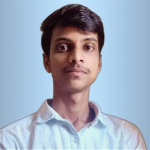
WRITTEN BY Aehteshaam Shaikh
Aehteshaam Shaikh is working as a Research Associate - Data & AI/ML at CloudThat. He is passionate about Analytics, Machine Learning, Deep Learning, and Cloud Computing and is eager to learn new technologies.
Comments