Voiced by Amazon Polly |
Overview
This blog will cover important tactics and industry best practices to maximize costs and fully utilize generative AI on Amazon Web Services. Let’s work through the nuances of resource allocation and budgeting to make sure your AI initiatives push the envelope and continue to be economically viable.
Pioneers in Cloud Consulting & Migration Services
- Reduced infrastructural costs
- Accelerated application deployment
Introduction
Generative AI projects are experiencing a surge in popularity due to their ability to unlock creative possibilities and solve complex problems.
As a result, the field is witnessing a rapid expansion in innovative applications and projects.
Importance of Effective Cost Management on AWS
Mastering cost management is paramount in the realm of Generative AI Projects on AWS. Efficient allocation of resources and budget optimization ensure that the transformative potential of generative AI is harnessed without unnecessary financial strain. As AWS offers a scalable infrastructure, understanding and implementing cost-effective practices enhance project sustainability and contribute to maximizing the return on investment. This blog series will delve into strategic insights to empower practitioners to achieve the delicate balance between innovation and cost efficiency within the dynamic landscape of generic AI on AWS.
Understanding AWS Pricing for AI Services
- Overview of AWS AI Services:
- AWS offers diverse AI services, including machine learning, natural language processing, and computer vision.
- Notable services include Amazon SageMaker for model training and deployment, Amazon Comprehend for language analysis, and Amazon Rekognition for image and video analysis.
- Pricing Models for AI Services:
- AWS AI services typically follow a pay-as-you-go pricing model, where you pay for the resources and computing power used.
- Pricing can vary based on factors such as the type of AI service, the volume of data processed, and the complexity of algorithms employed.
- Some services may have tiered pricing, offering cost advantages as usage scales.
- Factors Influencing Costs in Generative AI Projects:
- Model Training: Costs associated with training generative AI models can be significant, influenced by the size of the dataset, the complexity of the model, and the duration of training.
- Inference Costs: Deploying models for real-time predictions incurs costs, and the number of inference requests impacts overall expenses.
- Data Transfer: Moving large volumes of data in and out of AWS can contribute to costs, especially in generative AI projects with extensive data requirements.
- Storage Costs: Storing datasets, trained models, and other project related data in AWS repositories adds to the overall expenses.
Strategies for Cost Management
- Resource Provisioning and Optimization:
Rightsizing Instances:
- Tailor the computing resources to the actual needs of the generative AI workload to avoid overprovisioning and unnecessary costs.
- Choose instance types that match the computational requirements, ensuring optimal performance without excess capacity.
Spot Instances and Cost Savings:
- Leverage AWS Spot Instances for generative AI workloads that can tolerate interruptions.
- Spot Instances offer significant cost savings compared to OnDemand instances, making them a cost-effective choice for certain tasks.
- Data Storage and Transfer Considerations:
Efficient Data Storage Practices:
- Optimize data storage by selecting appropriate AWS solutions based on access patterns and performance requirements.
- Implement data lifecycle policies to manage data retention and reduce unnecessary storage costs.
Minimizing Data Transfer Costs:
- Keep data transfer costs in check by strategically placing resources in AWS regions, reducing cross-region data transfer.
- Utilize AWS Direct Connect or Amazon CloudFront for optimized and cost-effective data transfer solutions.
- Monitoring and Scaling:
Utilizing AWS Monitoring Tools:
- Leverage AWS CloudWatch and AWS CloudTrail to monitor generative AI workloads and track resource utilization.
- Set up alerts for any abnormal behavior or potential cost overruns.
Implementing AutoScaling Based on Demand:
- Implement autoscaling policies to adjust the number of resources dynamically based on workload demand.
- Autoscaling ensures that the infrastructure scales up during peak demand and scales down during periods of lower activity, optimizing costs.
Budgeting and Forecasting
- Setting Realistic Budgets for Generative AI Projects:
- Define clear and realistic budgets for generative AI projects by considering data size, model complexity, and computational requirements.
- Account for training and inference costs in the budgeting process to avoid surprises during different project phases.
- Forecasting Usage and Costs:
- Utilize AWS cost management tools and historical data to forecast usage patterns and associated costs for generative AI workloads.
- Factor in potential scalability needs and variations in data processing requirements when creating usage forecasts.
Conclusion
In conclusion, effective cost management for generative AI projects on AWS is a dynamic process that involves strategic budgeting, continuous optimization, and collaborative efforts between AI and finance teams. Organizations can balance innovation and financial prudence by adopting best practices, regularly revisiting cost strategies, and leveraging AWS tools, ensuring the sustainable success of their generative AI initiatives on the cloud platform.
Drop a query if you have any questions regarding Gen AI and we will get back to you quickly.
Empowering organizations to become ‘data driven’ enterprises with our Cloud experts.
- Reduced infrastructure costs
- Timely data-driven decisions
About CloudThat
CloudThat is a leading provider of Cloud Training and Consulting services with a global presence in India, the USA, Asia, Europe, and Africa. Specializing in AWS, Microsoft Azure, GCP, VMware, Databricks, and more, the company serves mid-market and enterprise clients, offering comprehensive expertise in Cloud Migration, Data Platforms, DevOps, IoT, AI/ML, and more.
CloudThat is recognized as a top-tier partner with AWS and Microsoft, including the prestigious ‘Think Big’ partner award from AWS and the Microsoft Superstars FY 2023 award in Asia & India. Having trained 650k+ professionals in 500+ cloud certifications and completed 300+ consulting projects globally, CloudThat is an official AWS Advanced Consulting Partner, AWS Training Partner, AWS Migration Partner, AWS Data and Analytics Partner, AWS DevOps Competency Partner, Amazon QuickSight Service Delivery Partner, Amazon EKS Service Delivery Partner, Microsoft Gold Partner, AWS Microsoft Workload Partners, Amazon EC2 Service Delivery Partner, and many more.
To get started, go through our Consultancy page and Managed Services Package, CloudThat’s offerings.
FAQs
1. How can I control costs during the training phase of a generative AI project on AWS?
ANS: – To control training costs, consider rightsizing instances, utilize spot instances for cost savings, and optimize algorithms. Monitor resource usage using AWS tools and adjust capacity based on actual needs.
2. What are the key considerations for minimizing data storage and transfer costs in generative AI projects on AWS?
ANS: – Efficient data storage practices involve selecting appropriate storage solutions and implementing data lifecycle policies. Minimize data transfer costs by strategically placing resources and leveraging AWS Direct Connect or Amazon CloudFront.
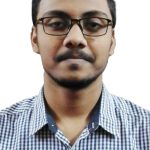
WRITTEN BY Aritra Das
Aritra Das works as a Research Associate at CloudThat. He is highly skilled in the backend and has good practical knowledge of various skills like Python, Java, Azure Services, and AWS Services. Aritra is trying to improve his technical skills and his passion for learning more about his existing skills and is also passionate about AI and Machine Learning. Aritra is very interested in sharing his knowledge with others to improve their skills.
Comments