Voiced by Amazon Polly |
Introduction
Amazon EMR is a comprehensive cloud platform for processing large-scale data sets, running interactive SQL queries, and deploying machine learning models. It utilizes popular open-source frameworks like Apache Spark, Apache Hive, and Presto.
This discussion will delve into active-active and active-passive DR approaches tailored for Amazon EMR, emphasizing scenarios involving Spark batch jobs that utilize external persistent storage, separate from the Amazon EMR infrastructure, and function with a solitary master node within the cluster.
Pioneers in Cloud Consulting & Migration Services
- Reduced infrastructural costs
- Accelerated application deployment
Prerequisites
It’s important to understand Amazon Managed Workflows for Apache Airflow (Amazon MWAA), a managed service for orchestrating workflows with Apache Airflow. Additionally, familiarity with Network Load Balancers is essential, as they distribute network traffic across multiple servers to ensure application reliability and performance.
- Solution overview
The following diagram illustrates the solution architecture.
Clients commonly utilize Amazon MWAA to dispatch Spark jobs to an Amazon EMR cluster through the Apache Livy REST interface. By setting up Apache Livy to connect with a Network Load Balancer’s hostname rather than the master node’s hostname of Amazon EMR, it eliminates the need for altering Livy connections in Amazon MWAA each time a cluster is initiated or terminated. For an active-active configuration, Network Load Balancer target groups can be associated with several master nodes of Amazon EMR clusters. Conversely, in an active-passive arrangement, a fresh Amazon EMR cluster can be spun up when a failure is detected, and its master node can be linked with the Network Load Balancer’s target group. The Network Load Balancer is designed to conduct regular health assessments and route traffic to operational targets, thereby maintaining uninterrupted operations even if an Amazon EMR cluster is compromised due to an Availability Zone disruption or other factors that impact the health of the cluster.
- Active-active DR strategy
An active-active disaster recovery configuration entails operating dual Amazon EMR clusters, each mirroring the other’s setup across separate Availability Zones. They can be initiated with the least necessary capacity to economize at the expense of running two concurrently active clusters. The managed scaling feature of Amazon EMR dynamically resizes the clusters in alignment with the fluctuating demands of the workload. It scales up by adding instances as required and scales down by terminating surplus instances post-task completion. This approach reduces the recovery time to virtually none, optimizing costs. It’s particularly beneficial for enterprises prioritizing continuous uptime and seamless failover capabilities for their data analytics operations.
With Amazon EMR managed scaling, clusters are designed to automatically modulate the count of instances or compute units in tune with the changing demands of the workload. Amazon EMR diligently observes cluster metrics to inform scaling decisions, ensuring a reasonable balance between cost efficiency and performance.
Network Load Balancer using the AWS Management Console
- Network Load Balancer using the AWS CLI (see Create a Network Load Balancer using the AWS CLI) or the AWS Management Console (see Create a Network Load Balancer). For this post, we will use the console.
- First, create a target group named emr-livy-dr and register the master IP addresses of both Amazon EMR clusters in this target group.
- To set up an internal Network Load Balancer that aligns with your Amazon EMR clusters, you’ll need to:
- Choose two distinct Availability Zones and their respective private subnets for the Network Load Balancer.
- Create a TCP listener on port 8998 (the default EMR cluster Livy port) to forward requests to the target group you created.
To ensure seamless integration between your Amazon EMR clusters and the Network Load Balancer, you’ll need to adjust the master security groups of the Amazon EMR clusters. Here’s how you can do it:
- Update the master security groups of the Amazon EMR clusters to permit ingress from the Network Load Balancer’s private IP addresses on port 8998.
- Search the elastic network interfaces using the Network Load Balancer’s name to locate the Network Load Balancer’s private IP addresses.
Once the target groups are verified as healthy, the Network Load Balancer will automatically route incoming requests on Livy port 8998 to the appropriate registered targets. This setup is crucial for maintaining uninterrupted access to the Amazon EMR clusters, especially when high availability and fault tolerance are essential.
- Obtain the DNS name of the Network Load Balancer
- We can also utilize an Amazon Route 53 alias record to route traffic to the Network Load Balancer’s DNS name using our domain name. This DNS name will be used in the Amazon MWAA Livy connection.
Set up and configure Amazon MWAA.
Complete the following steps
- Set up an Amazon MWAA environment in the same Region as your Amazon EMR cluster.
Include the following Python dependencies in the requirements.txt file and upload it to an Amazon Simple Storage Service (Amazon S3) bucket configured for DAGs:
1 2 3 4 |
apache-airflow>=2.1.0 apache-airflow-providers-http apache-airflow-providers-apache-livy[http] This installs LivyOperator, which we use in our DAG code. |
- If a Livy connection ID does not exist, create a new one.
- Here’s how you can utilize the provided sample DAG to submit a Spark application using LivyOperator, with the livy_default connection assigned to the livy_conn_id in the DAG code:
1 2 3 4 5 6 7 8 9 10 11 12 13 14 15 16 17 18 19 20 21 22 23 24 25 26 27 28 29 30 31 32 33 34 35 36 37 38 39 40 41 42 43 44 45 46 47 48 49 50 51 52 |
from datetime import timedelta, datetime from airflow.utils.dates import days_ago from airflow import DAG from airflow.providers.apache.livy.operators.livy import LivyOperator # Default arguments for the DAG default_args = { 'owner': 'airflow', "retries": 1, "retry_delay": timedelta(minutes=5), } # DAG configuration dag_name = "livy_spark_dag" # S3 bucket details s3_bucket_name = "artifacts-bucket" spark_jar_path = f"s3://{s3_bucket_name}/spark-examples.jar" # Define the DAG dag = DAG( dag_id=dag_name, default_args=default_args, schedule_interval='@once', start_date=days_ago(1), catchup=False, tags=['emr', 'spark', 'livy'] ) # Livy operator configuration livy_spark_task = LivyOperator( file=spark_jar_path, class_name="org.apache.spark.examples.SparkPi", driver_memory="1g", driver_cores=1, executor_memory="1g", executor_cores=2, num_executors=1, task_id="livy_spark", conf={ "spark.submit.deployMode": "cluster", "spark.app.name": dag_name }, livy_conn_id="livy_default", dag=dag, ) # Define task dependency livy_spark_task |
Conclusion
In this article, we’ve outlined various strategies and factors to enhance your disaster recovery (DR) planning when utilizing Amazon EMR on Amazon EC2, along with Network Load Balancer and Amazon MWAA. We have also detailed the procedures to establish the environments needed for an effective DR setup.
Drop a query if you have any questions regarding Amazon EMR and we will get back to you quickly.
Making IT Networks Enterprise-ready – Cloud Management Services
- Accelerated cloud migration
- End-to-end view of the cloud environment
About CloudThat
CloudThat is a leading provider of Cloud Training and Consulting services with a global presence in India, the USA, Asia, Europe, and Africa. Specializing in AWS, Microsoft Azure, GCP, VMware, Databricks, and more, the company serves mid-market and enterprise clients, offering comprehensive expertise in Cloud Migration, Data Platforms, DevOps, IoT, AI/ML, and more.
CloudThat is recognized as a top-tier partner with AWS and Microsoft, including the prestigious ‘Think Big’ partner award from AWS and the Microsoft Superstars FY 2023 award in Asia & India. Having trained 650k+ professionals in 500+ cloud certifications and completed 300+ consulting projects globally, CloudThat is an official AWS Advanced Consulting Partner, Microsoft Gold Partner, AWS Training Partner, AWS Migration Partner, AWS Data and Analytics Partner, AWS DevOps Competency Partner, Amazon QuickSight Service Delivery Partner, Amazon EKS Service Delivery Partner, AWS Microsoft Workload Partners, Amazon EC2 Service Delivery Partner, and many more.
To get started, go through our Consultancy page and Managed Services Package, CloudThat’s offerings.
FAQs
1. What is Disaster Recovery, and why is it crucial for Amazon EMR on Amazon EC2?
ANS: – For Amazon EMR on Amazon EC2, it’s essential because Spark workloads often handle large volumes of critical data, and any disruption can lead to significant financial and operational impacts. Being prepared ensures business continuity and minimizes the risk of data loss or service interruption.
2. What are some effective Disaster Recovery strategies for Spark workloads on Amazon EMR EC2?
ANS: – Effective strategies include implementing fault tolerance mechanisms within Spark applications, such as replication and checkpointing, to ensure data integrity and availability. Configuring automated backups of data stored on Amazon S3 and setting up standby clusters can facilitate quick recovery in case of failures. Regular testing and monitoring of these strategies are vital to ensure they function as expected during emergencies.
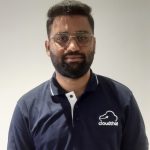
WRITTEN BY Sunil H G
Sunil H G is a highly skilled and motivated Research Associate at CloudThat. He is an expert in working with popular data analysis and visualization libraries such as Pandas, Numpy, Matplotlib, and Seaborn. He has a strong background in data science and can effectively communicate complex data insights to both technical and non-technical audiences. Sunil's dedication to continuous learning, problem-solving skills, and passion for data-driven solutions make him a valuable asset to any team.
Comments