Voiced by Amazon Polly |
Apache Spark has gained immense popularity for its ability to process large datasets quickly and efficiently. However, optimizing Spark jobs is crucial to fully leverage its potential and achieve high performance. In this blog, we will explore various best practices for optimizing Spark jobs, helping you improve execution speed, resource utilization, and overall efficiency.
Customized Cloud Solutions to Drive your Business Success
- Cloud Migration
- Devops
- AIML & IoT
Understanding Spark Architecture
Before diving into optimization techniques, it’s essential to understand Spark’s architecture. Spark operates on a master-slave model, where the Driver handles job scheduling, and Executors perform the actual computations. Spark jobs consist of Transformations (which create a new dataset from an existing one) and Actions (which trigger the execution of transformations).
Best Practices for Optimizing Spark Jobs
- Optimize Data Storage
Choose the Right File Format
Selecting an appropriate file format can significantly impact performance. Parquet and ORC are columnar formats optimized for read-heavy operations, providing better compression and faster query execution. If you’re working with large datasets, consider converting data to these formats.
Partitioning Your Data
Partitioning divides a dataset into smaller chunks, which allows Spark to process them in parallel. Choose partitioning columns wisely based on how data will be queried. For example, if you frequently filter by date, partitioning by date can enhance performance.
- Tune Spark Configuration
Memory Management
Memory is a critical resource in Spark. Adjust configurations such as spark.executor.memory and spark.driver.memory to allocate adequate memory for your executors and driver. Keep in mind that allocating too much memory can lead to garbage collection issues.
Executor and Core Configuration
The number of executors and cores assigned to your Spark job can affect performance. A common configuration is to set spark.executor.instances to a number that evenly divides your dataset size. Additionally, spark.executor.cores should be set according to the nature of the tasks too many cores can lead to contention, while too few can underutilize resources.
- Optimize Data Processing
Use DataFrames and Datasets
DataFrames and Datasets provide a higher-level abstraction compared to RDDs (Resilient Distributed Datasets). They come with optimized execution plans through Catalyst, Spark’s query optimizer. Use DataFrames or Datasets wherever possible for improved performance.
Minimize Shuffling
Shuffling occurs when data is redistributed across partitions, often leading to increased latency. To minimize shuffling:
- Use reduceByKey instead of groupByKey, as it performs the aggregation during the shuffle.
- Use partitioning and bucketing to control data distribution and reduce unnecessary shuffles.
- Broadcast Variables
When working with large datasets that need to be joined with smaller datasets, consider using broadcast variables. Broadcasting allows you to distribute the smaller dataset to all executors, minimizing data transfer overhead.
This approach speeds up operations by reducing the amount of data shuffled between nodes.
- Caching and Persisting Data
For iterative algorithms or jobs that reuse the same RDD or DataFrame multiple times, caching or persisting the data can lead to significant performance improvements. Use the persist() method to store data in memory, allowing quick access for subsequent actions.
Choose an appropriate storage level based on your memory constraints and application requirements.
- Optimize Joins
Joins can be expensive operations, so optimizing them is crucial. Consider the following strategies:
- Broadcast Joins: If one of the datasets is small enough, use broadcast joins to reduce shuffle.
- Skewed Joins: Identify skewed data (where one key has significantly more records) and handle it by salting keys or using techniques like repartitioning to balance data distribution.
- Leverage Window Functions
When performing calculations over a sliding window of data, use Spark’s built-in window functions instead of manual grouping and aggregation. Window functions are optimized and can lead to better performance.
- Monitor and Debug
Utilize Spark’s built-in web UI to monitor your job’s performance. The UI provides insights into stages, tasks, and their execution times, helping you identify bottlenecks. Additionally, leverage logs for debugging and to understand job behaviour.
- Use Efficient Serialization
Serialization can significantly impact performance, especially when shuffling data. By default, Spark uses Java serialization, which can be slow. Switch to Kryo serialization, which is faster and more efficient.
- Scale Appropriately
Finally, ensure that your Spark cluster is appropriately scaled based on your workload. If your jobs are consistently slow, consider increasing the number of nodes or upgrading your existing hardware to handle larger workloads efficiently.
Conclusion
Performance tuning is often an iterative process. Continuously monitor your Spark jobs, test different configurations, and adjust your strategies based on the workload and data characteristics. Optimizing Spark jobs requires a holistic understanding of both the framework and the data being processed. By following these best practices—optimizing data storage, tuning configurations, minimizing shuffling, and leveraging Spark’s powerful abstractions—you can significantly improve the performance of your Spark applications.
Get your new hires billable within 1-60 days. Experience our Capability Development Framework today.
- Cloud Training
- Customized Training
- Experiential Learning
About CloudThat
CloudThat is a leading provider of Cloud Training and Consulting services with a global presence in India, the USA, Asia, Europe, and Africa. Specializing in AWS, Microsoft Azure, GCP, VMware, Databricks, and more, the company serves mid-market and enterprise clients, offering comprehensive expertise in Cloud Migration, Data Platforms, DevOps, IoT, AI/ML, and more.
CloudThat is the first Indian Company to win the prestigious Microsoft Partner 2024 Award and is recognized as a top-tier partner with AWS and Microsoft, including the prestigious ‘Think Big’ partner award from AWS and the Microsoft Superstars FY 2023 award in Asia & India. Having trained 650k+ professionals in 500+ cloud certifications and completed 300+ consulting projects globally, CloudThat is an official AWS Advanced Consulting Partner, Microsoft Gold Partner, AWS Training Partner, AWS Migration Partner, AWS Data and Analytics Partner, AWS DevOps Competency Partner, AWS GenAI Competency Partner, Amazon QuickSight Service Delivery Partner, Amazon EKS Service Delivery Partner, AWS Microsoft Workload Partners, Amazon EC2 Service Delivery Partner, Amazon ECS Service Delivery Partner, AWS Glue Service Delivery Partner, Amazon Redshift Service Delivery Partner, AWS Control Tower Service Delivery Partner, AWS WAF Service Delivery Partner and many more.
To get started, go through our Consultancy page and Managed Services Package, CloudThat’s offerings
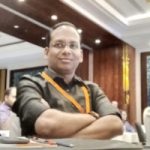
WRITTEN BY Nitin Kamble
Comments